Alexander Geiger, M.Sc.
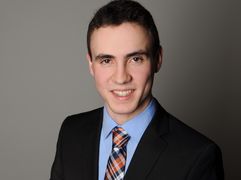
Room: Research group MITI, 1st floor
Trogerstraße 10, 81675 Munich
Current Projects
ManoVis
The subject of the ManoVis cooperation project is the development of optimised, multimodal analysis methods for diseases of the oesophagus. The focus is on the development of a diagnostic system that enables an optimal, functional and morphological evaluation of the oesophageal movement (motility). Up to now, the conventional approach to diagnosis has been to carry out various measurement methods (X-ray kinematography, pressure measurement, impedance measurement) one after the other. However, the direct temporal relationship between the individual measurements is lost, which reduces the diagnostic significance. The aim of the project is therefore the temporal synchronisation of the different modalities as well as the development of a fused visualization that combines all relevant partial information of the individual modalities and presents it in an intuitively understandable way. All information that is important for the diagnosis should be able to be grasped "at a glance". In a second step, it will be investigated to what extent X-ray kinematography can be replaced with the help of the available information from the other measurement methods. This could potentially significantly reduce the X-ray radiation that is harmful to the patient.
Publications
2024
- A. Geiger, L. Bernhard, F. Gassert, H. Feußner, D. Wilhelm, H. Friess, A. Jell, "Towards multimodal visualization of esophageal motility: fusion of manometry, impedance, and videofluoroscopic image sequences", Int J CARS, 2024, doi: 10.1007/s11548-024-03265-1
- A. Geiger, L. Wagner, D. Rueckert, D. Wilhelm, A. Jell, "Detecting and clustering swallow events in esophageal long-term high-resolution manometry", 2024 arXiv preprint, arxiv:2405.01126.
2021
- D. Liu, K. Veeramachaneni, A. Geiger, V. OK Li, H. Qu, "AQEyes: Visual Analytics for Anomaly Detection and Examination of Air Quality Data", 2021 arXiv preprint, arXiv:2103.12910.
2020
- A. Geiger, D. Liu, S. Alnegheimish, A. Cuesta-Infante and K. Veeramachaneni, "TadGAN: Time Series Anomaly Detection Using Generative Adversarial Networks", 2020 IEEE International Conference on Big Data (Big Data), 2020, doi: 10.1109/BigData50022.2020.9378139.