Laboratories
AI in Optoacoustics
The biomedical imaging workflow
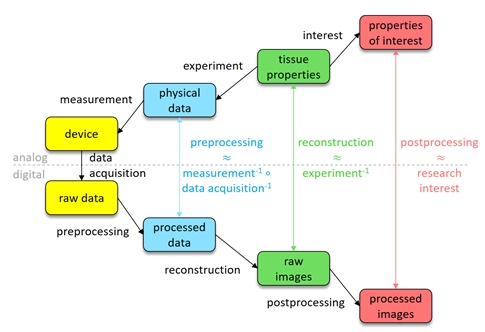
Research Outline
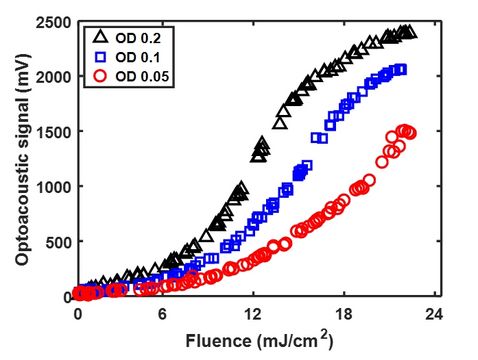
High-quality biomedical imaging needs mathematical reconstruction procedures that are accurate and efficient at the same time, and subsequent data analysis that is reliable and insightful.
At the group for mathematical modelling and inverse problems of CBI, we design innovative algorithms for biomedical imaging modalities based on sophisticated mathematical models. Our main focus is multispectral optoacoustic tomography (MSOT) and its combination with ultrasound imaging (OPUS). We also contribute to the analysis of the huge amount of data that is generated within the institute and in our research collaborations. Our goal is to drive the development towards quantitative optoacoustic and ultrasound imaging, thereby increasing the method's impact in translational medicine.
In close collaboration with the other groups at CBI - in particular with the group for machine learning in biomedical imaging and the group for multispectral optoacoustic tomography - we focus our research to the following three areas.
Mathematical modelling. In order to even formulate the problem of information extraction from imaging data, a proper model of the whole imaging process is necessary. To quantitatively and efficiently capture the different aspects of imaging, including the propagation of uncertainties, is the scope of mathematical modelling. In particular, in biomedical imaging, models for the probe, the optical and acoustic interaction of tissue and probe, the measurement, and the data acquisition are needed. Mathematics is not only the appropriate language for exact models, but provides diverse tools and concepts that allow to capture the validity of a model.
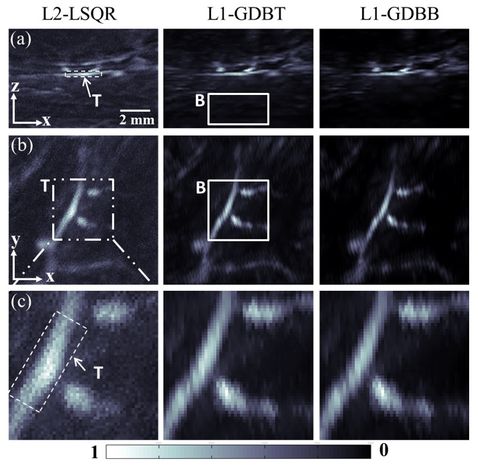
Inverse problems. The reconstruction of properties of interest from imaging data is an inverse problem, as it performs the inversion of a forward model of the imaging process. Abstractly, this problem can be divided into three steps: (1) preprocessing, which tries to approximate the physical response of the system by inverting data acquisition and measurement, (2) reconstruction, which tries to infer the tissue properties from the knowledge of the response by inverting the interaction, and (3) postprocessing, which extracts the properties of interest from the reconstruction. As most clinical applications ask for quantitative information, our goal is to not only retrieve the best possible estimate of the desired properties, but also to develop tools that reliably quantify the uncertainty of the reconstructions.
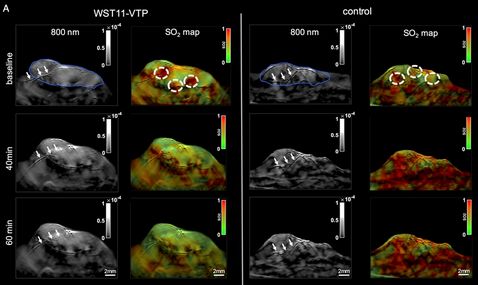
Data analysis. In order to extract clinically relevant information from the reconstructed data, it needs to be organized, structured, and analysed. Advances in computational science and data science, in particular in statistical learning, provide a rich toolbox for these purposes. We aim at further improving these methods and optimally adapting them to our needs. In addition, data can be used to improve the modelling and inversion steps by data-driven methods. For example, an efficient low-dimensional model for the class of structures of interest could be derived from the data.
Relevant publications
1. Jaya Prakash, et. al, "Maximum entropy based non-negative optoacoustic tomographic image reconstruction," IEEE Transactions on Medical Imaging (under review). arxiv: arxiv.org/abs/1707.08391
2. Volker Neuschmelting, et. al., "WST11 Vascular Targeted Photodynamic Therapy Effect Monitoring by Multispectral Optoacoustic Tomography (MSOT) in Mice," Theranostics (in press).
3. Jaber Malekzadeh, et. al., "Nonlinear optoacoustic readings from diffusive media at near infrared wavelengths," Journal of Biophotonics (in press).
4. Yiyong Han, et. al., "Optoacoustic reconstruction using fast sparse representation," Optics Letters 42(5), 979-982 (2017).
5. Almut Glinzer, et. al., "Targeting elastase for molecular imaging of early atherosclerotic lesion," Arteriosclerosis, Thrombosis, and Vascular Biology 37(3), 525-533 (2017).
6. Xiaopeng Ma, et. al., "Hybrid Fluorescence Molecular Tomography/X-ray Computed Tomography for imaging integrin expression in Non-Small Cell Lung Cancer," Neoplasia 19(1), 8-16 (2017).
7. Hailong He, et. al., "Optoacoustic tomography using accelerated sparse recovery and coherence factor weighting," Tomography 2(2), 138-145 (2016).
8. Xiaopeng Ma, et. al., "Assessment of Asthmatic Inflammation Using Hybrid Fluorescence Molecular Tomography - X-ray Computed Tomography," Journal of Biomedical Optics 21(1), 15009 (2016).
9. Ivan Olefir, et. al., "Hybrid multispectral optoacoustic and ultrasound tomography for morphological and physiological brain imaging,'' Journal of Biomedical Optics 21(8), 086005 (2016).
10. Stratis Tzoumas, et. al., ''Eigenspectra optoacoustic tomography achieves quantitative blood oxygenation imaging deep in tissues,'' Nature Communications 7, 12121 (2016).
11. V. Ermolayev, et. al., ''Early recognition of lung cancer by integrin targeted imaging in K‐ras mouse model,'' International Journal of Cancer 137(5), 1107-1118 (2015).
12. Markus T Berninger, et. al., ''Fluorescence molecular tomography of DiR-labeled mesenchymal stem cell implants for osteochondral defect repair in rabbit knees,'' European Radiology 27(3), 1105-1113 (2015).
13. Pouyan Mohajerani, et. al., ''Optical and optoacoustic model-based tomography,'' IEEE Signal Processing Magazine 32(1), (2015).
14. Pouyan Mohajerani, et. al., ''Model-based frequency domain optoacoustic tomography: theory and simulation results,'' Photoacoustics 2(3), 111-118 (2014).
15. Pouyan Mohajerani, et. al., ''FMT-PCCT: Hybrid fluorescence molecular tomography-X-ray phase-contrast CT imaging of mouse models,'' IEEE Transactions on Medical Imaging 33(7), 1434-1446 (2014).